Design of Experiments Online Course - DOE Training | GoSkills
Design of Experiments
Skills you’ll gain
This course is part of our Lean Six Sigma Black Belt program, which consists of eight courses designed to prepare you for the International Association of Six Sigma Certification (IASSC) Black Belt exam. We recommend you take all eight courses in the program to be fully prepared for the exam.
What you will learn
In this Design of Experiments online course, you will learn the Design of Experiments or DOE. This design technique, which can be applied in several different methods, takes the results from a few carefully designed experiments and uses those results to create equations that explain how the product, process or system works.
By the end of the course, you will know what the keys to a successful DOE analysis are, and you will be able to conduct a Full Factorial DOE and a Fractional Factorial DOE. If you are a member or leader of an analysis team using a methodology such as Lean Six Sigma, this technique will be a significant aid when your problem resolution requires a major change to your systems.
In addition to covering experimental design approaches and methodologies, you will learn to use popular statistical analysis application Minitab to conduct your DOE and apply your results.
The design of experiments technique is incredibly powerful when working with new products, new technologies, or when migrating an existing technology into a new application. It is also very helpful for identifying the critical few parameters that will drive the performance of the product, process, or system. When you are in a discovery mode of analysis, this technique provides a path to important insights.
Who this Design of Experiments online course is for
This course can be taken as part of the GoSkills Lean Six Sigma Black Belt training program, to prepare for certification with IASSC. It is also a good stand-alone course to improve proficiency and expand your skill set in any industry with responsibility for technology deployment or product and process development.
This course will be from the standpoint of helping you to make wise decisions about your product and process design and management, not conducting mathematical proofs or solving complex matrix mathematics.
Highlights:
- 24 practical tutorials with videos, reference guides, exercises and quizzes.
- Designed to prepare you in part for the IASSC Black Belt exam. To prepare in full, you should take all eight courses in our Lean Six Sigma Black Belt program.
- Identify when and why to do a DoE, and recognize the steps of the process.
- Understand the difference between the full factorial approach and fractional factorial approaches, and their pros and cons.
- Recognize when to use a Plackett-Burman and Taguchi DoE and how to design these types of study.
- Learn how to conduct DoE analysis in popular statistical analysis program, Minitab.
- Understand how design and problem solving teams can apply DOE results to make wise decisions.
- Master the key principles for success when conducting a DOE study.
- Gain critical skills for your role in technology deployment, product and process development, or Lean Six Sigma team.
- Earn 7 PDUs or contact hours toward your Project Management education for certification with PMI.
Once enrolled, our friendly support team and tutors are here to help with any course related inquiries.
Syllabus
Download syllabus-
1
Full Factorial DOE Methodology This lesson describes the eight steps to be followed when conducting a full factorial DOE. 6m
-
2
Factor Selection This lesson explains the different types of factors that are involved in the DOE study design including control factors and response factors. The characteristics that should be used when selecting each type of factor are discussed. 6m
-
3
Full Factorial DOE Study Design This lesson explains how to design the study so that the statistical analysis can be performed. The preparation of the test sample configurations is explained. The use of design features of replication, center points and blocking are also addressed. 6m
-
4
Conducting the Study This lesson addresses how to execute and control each of the experimental runs in the study. It also explains the importance of the measurement system that is used. 5m
-
5
DOE Functional Equation The statistical analysis of the full factorial DOE results in the determination of the coefficients for a design space equation that relates all the control factors to the response factors. This equation includes interaction effects between control factors. This equation can then be used by designers to solve for the best overall system performance. 9m
-
6
DOE in Minitab Minitab is the statistical analysis software application that is most often used with Lean Six Sigma projects. Minitab has a Wizard that guides you through the setup and design of a Design of Experiments study. This lesson demonstrates how to use that Wizard. 5m
-
1
Fractional Factorial Pros and Cons This lesson compares the difference between the full factorial approach and fractional factorial approaches. It explains the pros and cons of using a fractional factorial methodology. 6m
-
2
Fractional Factorial DOE Methodology This lesson describes the nine steps to be followed when using one of the fractional factorial DOE methods. The emphasis is one how the steps differ from the full factorial DOE methodology. 6m
-
3
Confounding Effects This lesson explains the importance of designing a fractional factorial DOE study using a set of experiments that is balanced and orthogonal. Otherwise the runs can become confounded and that will invalidate the statistical analysis of the results. 5m
-
4
Factor Selection This lesson builds on the previous factor selection lesson. However, now it addresses how the factor selection process changes as a fractional factorial DOE progresses through two or three levels of studies. 5m
-
5
Plackett-Burman DOE The Plackett-Burman DOE is a special case fractional factorial DOE. It is used as a screening study when there are a large number of control factors. This lesson explains when to use Plackett-Burman DOE and how to design this type of study. 4m
-
6
Taguchi DOE The Taguchi DOE is a special case fractional factorial DOE. It is used primarily for analyzing manufacturing processes. The Taguchi DOE separates the control factors into two categories and analyzes them with different DOE approaches. This lesson explains the characteristics of this type of study. 7m
-
1
DOE Analysis in Minitab This lesson reviews the different types of graphical and tabular results for a DOE study that are generated by Minitab. Each of these types of results provides a different perspective on the analysis of the design that is being studied. 5m
-
2
DOE in Design Creation The DOE results can be used by design teams to make wise design decisions. This lesson will address how to use the DOE results in predicting system performance, designing system controls and establishing tolerances on system control and response factors. 5m
-
3
DOE in Design Optimization The DOE results can be used by design teams to improve and optimize an existing design based upon new needs or uses. The structure of the DOE study, particularly the fractional factorial DOE methodologies, allows the design team to easily establish optimal performance in a variety of settings. 6m
-
4
DOE in Problem Solving The DOE results can be used by problem solving teams, such as Lean Six Sigma project teams, to identify which factors provide the major contribution to the problem or problem performance. It can also be used to explain the expected benefit from implementing different types of solutions. 4m
-
5
DOE Keys to Success This final lesson reviews the key principles that must be followed when conducting a DOE study. It highlights the benefit of each and the dangers if the principle is not properly applied. 7m
-
6
DOE Factorial Plots One of the most common techniques for analyzing the results of a DOE study in Minitab is to review the factor plots. These will provide insight into the optimal settings for control factors. The interactive plots will also highlight the settings associated with local maximum or minimum performance levels. 5m
-
7
Path of Steepest Ascent/Descent Some DOE analyses will indicate that the optimal performance of the system would occur when control factors are set beyond the bounds of the study. When this occurs, it is best to shift the study to the likely region of optimal performance and then determine the best control factor settings. Following the path of steepest ascent or descent will ensure that the new analysis is conducted in a region with maximum or minimum performance. 7m
Certificate
Certificate of Completion
Awarded upon successful completion of the course.

Instructor
Ray Sheen
Ray is a certified Project Management Professional (PMP) with the Project Management Institute, a certified Scrum Master with Scrum Alliance, and certified Lean Six Sigma Black Belt with IASSC. He is a member of the Project Management Institute and the Product Development Management Association.
He is president and founder of Product & Process Innovation, Inc. and is a veteran business leader with over 25 years of executive, project management, and engineering management experience. Ray has worked in several industries including aerospace, electrical distribution and utilities, biotechnology, appliances, electronics, machining, medical devices, pharmaceutical, automotive, and financial services. He has held executive management positions in a Fortune 500 company and has been involved in entrepreneurial startup organizations.
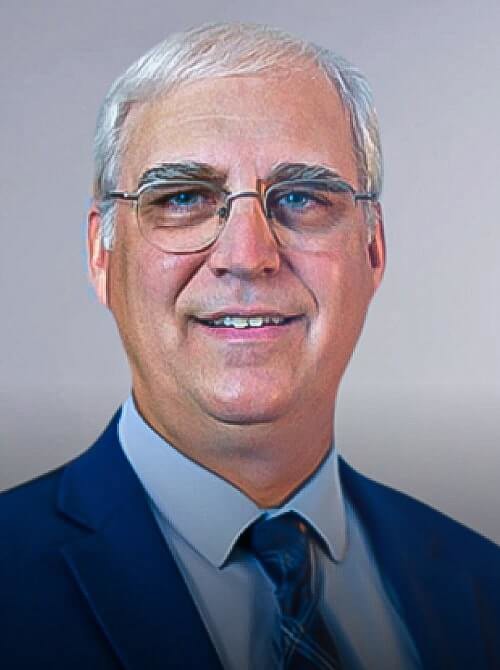
Ray Sheen
Project Management Instructor & Author
Accreditations
Link to awards GoSkills Ltd is an IASSC Accredited Training Organization™. The PMI ATP seal, PMI, PMP, CAPM and PMBOK are registered marks of the Project Management Institute, Inc.How GoSkills helped Chris
I got the promotion largely because of the skills I could develop, thanks to the GoSkills courses I took. I set aside at least 30 minutes daily to invest in myself and my professional growth. Seeing how much this has helped me become a more efficient employee is a big motivation.
